Structural Stability in Machine Learning
Seminar organized by OptimiX
Presenter
Vassilis DigalakisAffiliation
HEC Paris
Date
November 14, 2024 at 14:00
Local
Flajolet room
Abstract:
Stability in machine learning (ML) is important for ensuring consistent performance and enhancing interpretability. For example, in healthcare, patient mortality risk prediction models may change upon retraining with new batches of data, but are likely to do so in a “smooth” way; in sustainability, the same holds true for energy consumption prediction over consecutive hours of the day or air quality prediction over adjacent spatial areas. Abrupt changes in a model’s structure or the resulting analytical insights can lead to hesitation for adoption. In this talk, I will present my research on stable ML through the lens of industry collaborations in healthcare and sustainability. First, I will introduce the framework of slowly varying regression under sparsity, allowing sparse regression models to exhibit controlled variations under some temporal, spatial, or general graph-based structure. Assessing stability in decision trees presents new challenges compared to regression models: to address this, I will propose a novel distance metric for decision trees and use it to determine a tree’s level of stability. Finally, I will present a model-agnostic framework to stabilize interpretable models’ structures or black-box models’ insights upon retraining with new data. We have tested the proposed methodologies on numerous real-world case studies and have shown that a controlled—and often negligible—decrease in predictive power can significantly improve the models’ stability and interpretability.
Bio:
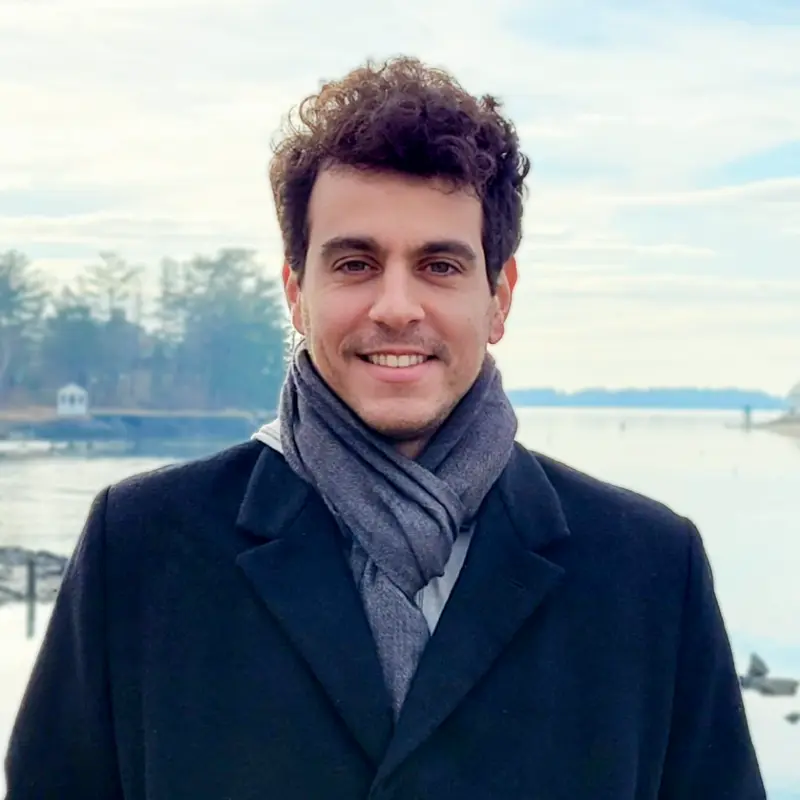